Drowning in big data?
In a world of ever growing data, enterprises, businesses, and entrepreneurs are relying far less on gut, intuition, and uninformed risk-taking to deliver results. Countless forms of big data and business-intelligence technologies have surfaced over the past decade, creating an explosion in increased analytics efficiency but also a human-resource paradigm.
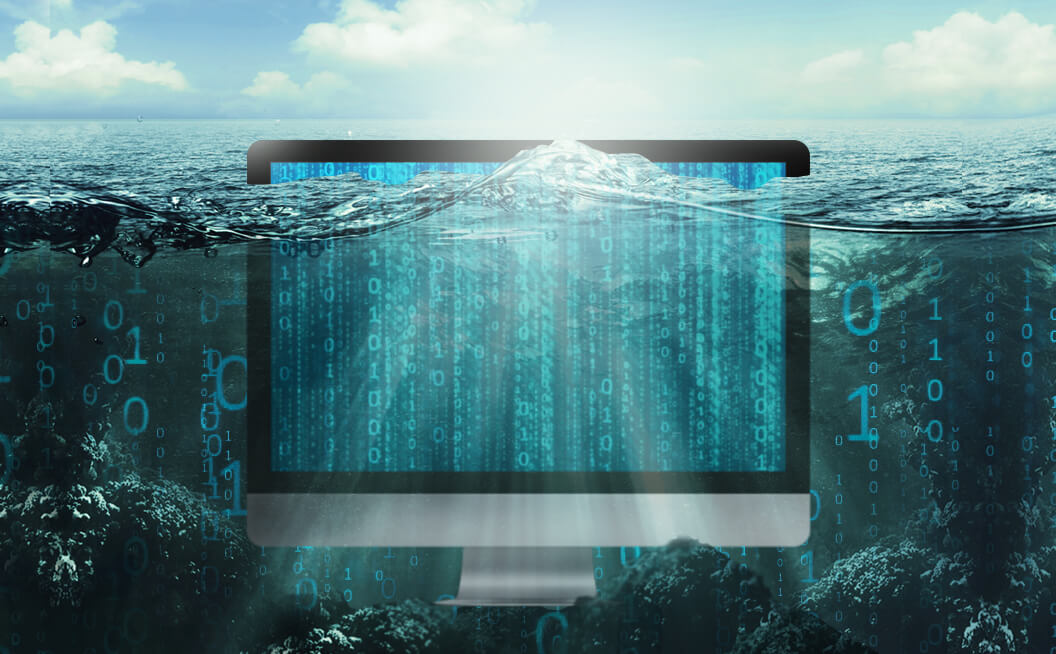
Democratization of machine learning and artificial intelligence has acted as a catalyst to this rapid change, allowing for more sophisticated and automated forms of insights and intelligence. However, many of these technologies still struggle with creating the best possible experience around their respective big-data silos. There is no question that we “crunch” any and all types of data, but we fail to deliver those insights in a highly digestible, actionable, and interpretable way.
The human mind cannot and will not adapt or perform at the pace of our technological advancements, so as an industry, we must focus aggressively on creating more intuitive and simplistic ways to interpret the outputs of our exponentially complex technologies. With an increase in complexity comes the need for enhanced simplicity. In stating this, few of our technologies employ a user experience on par with the sophistication of the underlying intelligence and product architecture. As UX/UI designers, we must think relentlessly to create products that can deliver true value and user efficiency on the front end. Many end users still struggle with the most optimal way to uncover these insights and, more importantly, take meaningful action on them.
Ironically, this article is an attempt to forecast the industry’s movement away from the “one-size-fits-all” big-data approach. Many of industries technology’s claim to fame is the ability to perform and create analysis on any and all types of data related to a particular enterprise or business. Companies like Domo and Tableau attempt to be “everything for everyone” and this is quite possible from an intelligence and machine learning perspective however the real caveat is creating an effective experience around this much data diversity and the resulting, “necessary functionality”. For this reason smaller, more nimble big-data startups are taking a more customized approach to design technologies with an experience that preserves value for the end user while mitigating interpretation dilution.
Over the next decade, we will see the big-data industry divide itself into more sophisticated forms of products with emphasis on targeted purposes and functions. For example, the backbone technology for growing retailers should not and will not be the same technology used to operate and scale a manufacturing business. Companies that choose to leverage the “one-site-fits-all” technologies will most likely fall behind their competitive set as their systems hit the inevitable intelligence ceiling created by feature and experience bloat, causing further performance disparity over time.
Until machines begin making decisions rather than supporting them, there will be a critical need for better, more intuitive interfaces and experiences. We should not concern ourselves with creating increasingly sophisticated forms of intelligence, as this will come with the democratization of artificial intelligence and the growing liberty in utilizing it. Our current responsibility as big-data technologies is to begin emphasizing what the actual product solves for and how it presents this.
Recommended Posts